Speech by Christopher Woolard, Executive Director of Strategy and Competition at the FCA, delivered at The Alan Turing Institute's AI ethics in the financial sector conference[1].
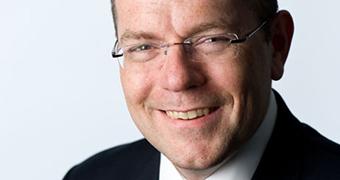
christopher woolard.jpg
Speaker: Christopher Woolard, Executive Director of Strategy and Competition
Location: The Brewery, London
Delivered on: 16 July 2019
Highlights:
- As the regulator, we consider the use of AI in financial services from three main perspectives.
- Firstly, which parts of the debate are novel and where is there continuity.
- Secondly, how can we ensure AI is creating value for citizens.
- And lastly, how can we work with others to develop a shared understanding that will determine our approach over the years ahead.
Note: this is the speech as drafted and may differ from the delivered version.
Introduction
Good morning. It is a pleasure to be here with The Alan Turing Institute to talk about artificial intelligence (AI) and how we, as the regulator, view its application in financial services.
In Ian McEwan’s latest book, Machines Like Me, we are presented with a vision of the 1980s in which Alan Turing has reached old age and life-like humanoid AIs are available for purchase.
The protagonist, Charlie, buys Adam, a cutting-edge android, believing he’s gaining a ready-made friend and companion. Yet what ensues is a love triangle between Adam, Charlie and Charlie’s girlfriend Miranda.
McEwan’s novel leaves the reader wondering if the ingenuity that created Adam is 'the triumph of humanism – or its angel of death'?
It’s a question we see played out in the news every day.
Whether it’s air safety, the use of facial recognition in policing or driverless cars, to quote McEwan: 'We are in the process of handing over responsibility for safety, but also for ethical decisions, to machines'.
These issues are ubiquitous to society as a whole, but take on a particular resonance in finance. Finance plays a fundamental role at the heart of daily life for almost every single person. And how AI plays out here may determine how citizens feel about new technologies across the board.
Ultimately, there’s one question we have to ask: can decisions that materially affect people’s lives be outsourced to a machine?
And what might that mean for the future of regulation?
Today I want to talk about how we go about approaching that question as the regulator.
For us, there are three main aspects:
- continuity (which parts of this debate are genuinely new and which aren’t)
- public value (how we can create value for citizens)
- collaboration (how we’re working with others to answer the questions AI poses)
Continuity
Let’s start with continuity.
Superficial debate around AI and machine learning often descends into talk of robot armies and a dystopian decline in human agency.
But are we really living through a crisis of algorithmic control? The answer, at least when it comes to the use of AI in financial services, is – not yet.
We recently carried out a joint survey with the Bank of England to assess the current state of play.
What we found was that the use of AI in the firms we regulate is best described as nascent. The technology is employed largely for back office functions, with customer-facing technology largely in the exploration stage.
By and large those who lead financial services firms seem to be cognisant of the need to act responsibly, and from an informed position.
Rightly, the lessons of the crisis seem to be playing on industry minds. Too much faith was put in products and instruments that weren’t properly understood. Certainly, there is no desire to reverse progress on rebuilding public trust.
Perhaps unsurprisingly, the picture varies depending on the firm in question. Some larger, more established firms are displaying particular cautiousness. Some newer market entrants can be less risk averse. Some firms haven’t done any thinking around these questions at all – which is obviously a concern.
There is a balance to be struck here.
While awareness of regulatory and consumer risk is welcome, we don’t want this to act as a barrier to innovation in the interests of consumers.
For example, in the latest cohort of our regulatory sandbox, we’ve seen a number of tests relating to digital identity. Such propositions, which often use machine learning, help businesses verify the identities of their customers digitally, bypassing the need to go into a branch and have a cashier check whether your ID is genuine.
That’s both potentially good for competition – by reducing friction – but also may be more effective if sophisticated techniques can be deployed.
It’s worth reflecting on how emergent technologies today fit into the longstanding tradition of innovation in financial markets. After all – we’ve seen technical approaches to trading since the liberalisation of the 1980s.
Some areas, like algorithmic trading, are already pretty well developed. The use of algos here presents very specific threats – such as the challenge of flash crashes.
But the risks presented by AI will be different in each of the contexts it’s deployed. After all, the risks around algo trading will be totally different to those that occur when AI is used for credit ratings purposes or to determine the premium on an insurance product.
The FCA doesn’t have one universal approach to harm across financial services – because harm takes different forms in different markets and therefore has to be dealt with on a case by case basis.
And this will be the same with AI.
Higher level principles – such as transparency and accountability – are useful in providing a framework.
But in practice, we will have to consider the specific use case, identify the specific harms and then determine the specific safeguards needed.
One important safeguard will be governance.
As we’ve seen across financial services as a whole over the last decade, culture and governance matters when it comes to outcomes.
If firms are deploying AI and machine learning they need to ensure they have a solid understanding of the technology and the governance around it.
This is true of any new product or service, but will be especially pertinent when considering ethical questions around data. We want to see boards asking themselves: 'what is the worst thing that can go wrong' and providing mitigations against those risks.
Public value
While the issue of governance will hopefully be a familiar one to the firms we regulate, the question of what this looks like in practice for AI is novel.
There’s growing consensus around the idea that algorithmic decision-making needs to be ‘explainable’.
For example, if a mortgage or life insurance policy is denied to a consumer, we need to be able to point to the reasons why.
But what level does that explainability need to be? Explainable to an informed expert, to the CEO of the firm or to the consumer themselves?
When does a simple explanation reach a point of abstraction that becomes almost meaningless – along the lines of ‘we use your data for marketing purposes’ or ‘click here to accept 40 pages of small print’.
The challenge is that explanations are not a natural by-product of complex machine learning algorithms. It’s possible to ‘build in’ an explanation by using a more interpretable algorithm in the first place, but this may dull the predictive edge of the technology.
So what takes precedence – the accuracy of the prediction or the ability to explain it?
These are the trade-offs we’re going to have to weigh up over the months and years ahead.
That’s why we’ve partnered with The Alan Turing Institute to explore the transparency and explainability of AI in the financial sector. Through this project we want to move the debate on – from the high-level discussion of principles (which most now agree on) towards a better understanding of the practical challenges on the ground that machine learning presents.
We’ll be undertaking a joint publication around these themes, with a workshop planned for early next year.
While the widespread use of AI presents us with complex, ethically-charged questions to work through, it also holds enormous promise.
We’re already seeing its potential play out in areas like financial crime. Distinctive patterns and data typologies are now being identified by today’s machine learning tools.
And in retail banking – a utility of central importance to consumers that has long been bedevilled by a lack of innovation – AI has the ability to be genuinely transformative.
The implementation of Open Banking last year heralded the beginning of what is likely to be a period of profound evolution in the banking sector.
Its power lies in the opening up of data. By allowing consumers access to their information, they can better compare offerings from different providers. This in turn encourages the development of new business models offering innovative services, stimulating competition.
In this way, Open Banking addresses some of the asymmetry of power that exists between consumers and their banks.
This has big implications for AI. We all know that with access to the rich datasets facilitated by Open Banking, the potential for AI for the good of consumers is huge.
It would be premature to comment on the success of Open Banking at this early stage. But initial indicators are positive – with 100 regulated providers involved as of January this year. We remain optimistic that it has the potential to solve the perennial problem of weak competition in retail banking.
There are, however, some big caveats.
Exciting though innovations like Open Banking are, they don’t exist in a vacuum.
Technology relies on public trust and a willingness to use it. The public needs to see the value data can create for them.
The Facebook/Cambridge Analytica incident last year struck a heavy blow against consumer trust in data sharing, that is still playing out.
As we’ve said previously, a key determinant of future competition will be whether data is used in the interests of consumers or used by firms to extract more value from those consumers.
As the market in data grows and machine learning continues to develop, firms will find themselves increasingly armed with information and may be tempted into anti-competitive behaviours.
How does industry ensure it stays on the right track? The key is, as so often, customer-centricity.
Not only thinking about the problem technology is there to solve, but also whether the solution it offers involves acceptable trade-offs.
At a basic level, firms using this technology must keep one key question in mind, not just ‘is this legal?’ but ‘is this morally right?'
As regulators, we have a range of powers and tools to tackle these issues now, but as we see greater and greater use of technology, those tools may need to be updated for a fully digital age.
This is something we will be thinking about in our own work on the ‘Future of Regulation’, which I’ll return to shortly.
Collaboration
I know from my own conversations with firms that industry is already doing a lot of work around the ethical and moral questions posed by AI – a testament to the UK’s role in leading standards on machine learning globally.
But the rapid development of AI undeniably raises questions to which we don’t yet have answers – transparency and explainability chief amongst them.
It’s crucial that we engage with these issues now, not least because we expect the application of machine learning in financial services to increase substantially over the next few years.
This is going to require a combined effort. We – regulators, academics, industry and the public – need to work together to develop a shared understanding that will determine our approach over the years ahead.
I’ve already mentioned the work with The Alan Turing Institute on explainability.
At an international level, the FCA is also leading a workstream on machine learning and AI for IOSCO, exploring issues around trust and ethics and what a framework for financial services might look like.
As well as external engagement, we’re also looking inwards and asking whether there’s anything we can do differently as a regulator to ensure we’re ready for the challenges of the future.
AI is an excellent example of the huge waterfront the FCA is responsible for – and one that is only growing as financial markets continue to innovate.
At the same time, public expectations of us are changing. Post-crisis, there are very strong public demands for greater protection.
And playing out in the background are wider socio-economic trends which are influencing the landscape in which we work – an ageing population and the greater onus on consumers to manage their financial futures amongst them.
In the midst of this complex picture, where our responsibilities are growing, we need to think about how we can ensure our interventions have the biggest impact – how do we get the biggest bang for our buck with finite resources?
As part of that, we are taking a fundamental look at how we carry out the task of conduct regulation, and how we shape the regulatory framework going forward, in what we are calling our ‘Future of Regulation’ project.
This involves taking a fresh look at some of the core components[2] that determine our approach, including reviewing our Principles for Businesses and considering how we can become a more outcomes-based regulator.
We will also be liaising with the Treasury as part of the review announced in the Chancellor’s Mansion House speech on the way regulators coordinate their work.
There are big and complex questions to answer here, which go beyond the day-to-day operations of the FCA and other regulators. Chief amongst them is: how can we ensure the regulatory framework adapts to the changing economic, demographic and political environment in which it operates?
These issues need to be debated fully and openly. So a key part of our work will be to convene a wide-ranging conversation with our stakeholders about what the future of regulation should be.
In navigating these complex debates, our guiding principle is public value – where can we intervene to ensure the optimal balance of costs and benefits to society?
New technologies offer potential solutions to this end. Machine learning to identify suspicious transactions in real time is just one example.
But these innovations can’t be developed in isolation. The problems AI and machine learning have the potential to solve are cross-border, cross-sector, sometimes cross-agency.
So in order to thrash out an approach that delivers the maximum public value, we all have to put our heads together, collaboratively and internationally.
Conclusion
AI and its application in financial services is causing us to ask big questions – and the answers we arrive at have the potential to fundamentally alter society and the established order.
We can’t arrive at these answers on our own – the ramifications are too wide reaching – but as the regulator of one of the world’s biggest financial centres, we believe we have a key role to play.
We won’t shy away from that responsibility. We will look at the facts, examine the evidence and seek to shape an approach to AI that benefits the consumers that the financial system is ultimately there to serve.
It’s tempting to succumb to the panic over the emergence of an army of Adams in financial services.
But let’s keep one thing in mind.
The key to moving this debate on, and unlocking the benefits of AI for consumers, is real case studies and practical approaches.
That means considering AI in the context it’s actually used.
Facets of this debate are new and unprecedented. But there is continuity and our current rules are sufficient – for now.
There is an opportunity to come together and think about what kind of AI regime we actually want. I believe this point is now.
To end with a quote from Alan Turing himself: 'We can only see a short distance ahead, but we can see plenty there that needs to be done.'
I look forward to working with you to deliver it.