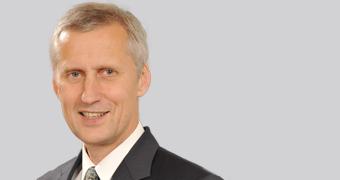
Speech by Martin Wheatley, Chief Executive of the FCA, at The Institute of Directors, London. This is the text of the speech as drafted, which may differ from the delivered version.
Content
The shift in Behavioural Economics
Price cap analysis: The technological empowerment of regulators
The shift in Behavioural Economics
In the interests of full disclosure – a topic I’ll come on to later – it’s worth starting by saying I’m not an economist by degree, more by occupation. In fact, I studied philosophy at university. Something I never thought I’d return to as I became, over the years, first an accountant, then a master of business, and finally a regulator. I find myself, however, more often than I ever thought possible, returning to questions of ethics, of fairness, of power and knowledge mismatches.
Having said that, I do want to argue this evening that the use of economics by regulators is more significant than ever to the future of the financial sector. So, it’s a great pleasure and privilege to be asked to deliver one of this year’s Beesley Lectures.
It’s also important in terms of timing. It is now almost exactly six years to the day since world leaders convened in Washington for the first G20 summit. Just two months after Lehman’s filed for Chapter 11 bankruptcy. For policy makers in regulation, the period that followed was clearly very challenging, with the immediate priority for economists one of reducing network risk.
All the policy talk pre-crisis had been on the importance of liberalising markets. Letting the invisible hand do its job, if you like, with exits for poor products and profits for good ones. Post crisis, the key question was why these cases seemed to defy that logic? Why did the market do its job very effectively in many areas – yet fail to do so in others
Today, those same prudential debates clearly remain important for leaders in both regulation and politics. But what I think is enormously significant in terms of moving things forward, is the fact that economic policy making is now every bit as crucial in the conduct space as the prudential space.
So, over the last three years there’s been significant (and much-needed) debate among economists over why products like PPI in the UK, currency swaps in Korea, mini-bonds in Hong Kong and so on and so forth, were able to scale up in to such significant events.
All the policy talk pre-crisis had been on the importance of liberalising markets. Letting the invisible hand do its job, if you like, with exits for poor products and profits for good ones. Post crisis, the key question was why these cases seemed to defy that logic? Why did the market do its job very effectively in many areas – yet fail to do so in others? Why did these products make so much money? How, in a rational market, could a product like PPI – with premiums adding 20% to the cost of a loan and a claims ratio of around only 16% – have sold in the numbers it did, and to people who couldn’t claim on them?
And, crucially, what do the characteristics common to each of these cases, tell us? The high profit margins that encouraged firms to market these products aggressively. The high levels of complexity in each instance. And, of course, the seemingly ubiquitous susceptibility of consumers to context and product framing – as well as industry understanding of this susceptibility (in Hong Kong I saw firms sending out marketing fliers for structured products on red paper – for luck – with pots of gold on them).
These are the kinds of questions that have, over time, become increasingly important to the future of our financial sector.
So, six years on from that first G20 conference and the beginning of all this critical analysis, what have those debates actually achieved in the conduct space?
The answer, in the UK at least, is a significant intellectual distancing from interventions that rely on self-stabilisation, equilibrium and efficiency in the financial markets. The arch-rationality of consumers.
Instead we’re enhancing traditional economic analysis by integrating it with behavioural techniques. Considering the demand-side as well as supply-side of competition – how real people interact with markets.
So, where ten years ago the solution to information asymmetries say, would have been to produce more disclosure that people either ignored or didn’t understand, today we’re designing policy based on how people engage with financial products.
For policy makers, this is important for two reasons. First because it has the potential to materially improve consumer outcomes. Second, because it can potentially increase competitive pressure on incumbents by reducing barriers to contestability like complexity, consumer inertia and so on. The argument being that firms have to work harder to retain their market share. Now, this might sound a small step to some. But for the financial industry and its customers, it is a potentially giant leap forward.
In the UK alone, there were some 18 separate competition reviews launched into retail banking between 2000 and 2010, starting with the Cruickshank Report. Yet if you look at ‘best buy tables’ today across a range of domestic debit and credit products, you invariably see the big players picking up high volumes of sales (often in markets where customer service does not seem to be an important consideration) despite quoting prices that aren’t market leading.
The obvious questions here: why are we still seeing these apparent breakdowns in price efficiency and rationality so often? To what extent are incumbents raising price above marginal cost? And, when they are, why are they not being disciplined by customers? And it’s this third, crucial, debate that is now the major imperative for financial regulators. So, instead of relying on the invisible hand to shape our interventions we lean against the drivers of irrationality.
In practice, this means regulators taking far greater account of the buy and sell-side issues that create PPI-type problems. Drip pricing; under-emphasised fees; reference pricing; under confidence and over confidence as well as specific behavioural economic issues like bounded rationality, which show that when people are presented with complex, difficult maths or many different moving parts, the norm is for them to make decisions that aren’t in their best interests.
In other words, people aren’t very good at making on-the-hoof calculations of probability or at assessing risk. So we make mistakes. In the words of Daniel Kahneman, we’re bad ‘intuitive statisticians’. We believe in the pot of gold.
An example from my previous role in Hong Kong. There, individuals who had term deposit accounts maturing were invited to meet the bank manager – the banks would then offer them a new deposit rate paying 1% or an alternative ‘safe’ product paying 7%.
‘Why does one pay 7%?’ was the question that consumers didn’t ask. They didn’t because it was offered to them by their bank and they trusted that the bank wouldn’t sell them a ‘risky’ product.
Had they known the 7% product was a complex structured product that was effectively writing credit insurance, they might have thought twice. But the product was so opaque consumers didn’t know the right questions to ask.
Why is there particular confidence today among economists that we can, this time, improve consumer outcomes and stimulate more effective competition? The answer is in technology, data and advances in economic techniques, which allow us to test behavioural interventions with much greater confidence and integrity than ever before.
Data and processing power
Now, these are clearly long-run, complex issues and behavioural economics is not particularly new. So, why is there particular confidence today among economists that we can, this time, improve consumer outcomes and stimulate more effective competition? The answer is in technology, data and advances in economic techniques, which allow us to test behavioural interventions with much greater confidence and integrity than ever before.
In fact, I want to argue tonight that it’s the specific combination of behavioural science, data and technology that has turned economics in to such an important feature of conduct regulation.
A key point here: nudges and the like can be very powerful, but they are not always a practical replacement for rules. Nor are they straightforward to apply, interventions that seem common sense in one area, don’t necessarily translate across to other markets or demographics.
So, in the UK for instance, the Government’s Behavioural Insights Team found they could improve the number of people who paid their taxes on time from some 68%, to 83%, by making non-payers aware that their neighbours were completing their returns.
In total, the team sent some 140,000 letters to taxpayers. Some control letters, so with no reference to social norms. Some with a statement that nine out of ten people in Britain pay their taxes on time. Some referencing specific response rates in the recipient’s local area. The latter letters were particularly effective, so social norms clearly worked powerfully here.
Yet when the same team tested the use of social norms to nudge people to sign the organ donor register, the effect was far more nuanced. Mentioning that thousands of people sign up every day increased the response from 2.3% to 2.9%. But combining this information with a picture of a group of people reduced it to 2.2%.
Now, in financial services this is a familiar concern of course. Regulators have been criticised before over the ineffectiveness of interventions, or the impact of unintended consequences. Behavioural changes in complex markets being especially difficult to predict.
In part, this was an inevitable consequence of wider technological limitations. 20 or so years ago, most of us didn’t have mobile phones – let alone access to the kind of data we do today. Google’s Eric Schmidt famously estimated we now create as much information every two days on the internet as was produced in the entire history of mankind up until 2003.
Instead, policy makers relied on relatively unsophisticated techniques like focus groups to test interventions. So, for example, in 1995 the UK’s Personal Investment Authority, as it was then, introduced a Key Features Documents, designed to give consumers clear product information and encourage them to shop around. That didn’t work. Most consumers didn’t look elsewhere. In fact, most only saw one Key Features Document per product – the one they bought.
So our predecessor, the FSA, sensibly shortened, improved, and standardised them, based on interviews and focus groups with consumers, and then re-branded them: ‘keyfacts’ documents.
The result? Consumers still didn’t use them to shop around. They liked having them, but saw them as a post-sale reference document – which is useful, yes, but frankly not the purpose they were designed for (one of the reasons we’re looking at them again). And that, ultimately, is why sophisticated field-testing, trials and big data analysis is now dominating so much of the FCA’s work across key areas like the pay-day price cap analysis – and competition activity in markets like cash savings and overdrafts.
In other words, instead of relying on intuition and guesswork, we combine trials, behavioural economics, and competition analysis, to work out what’s going on in each market – real markets, not just theoretical constructs.
Whether you agree with the fundamental principle of price caps or not, it’s frankly impossible to argue we’re not better placed to set them today than we were five years ago.
Price cap analysis: The technological empowerment of regulators
Our price-cap analysis work is a particularly good, and topical, example here of how far and fast the world has moved since my own generation entered financial services in the Thatcher-era. Today, regulators use vastly more advanced technology than Neil Armstrong used to reach the moon. And this processing power has been matched by a similar scaling up in the amount of information we have at our disposal. Big data, if you like.
So, during the price cap analysis work, using two specialist standalone computers – with 32 ‘hyperthreaded’ cores each and 12-additional core machines (for the technology-minded here) – we modelled cost, revenue and repayment information for some 2.3m anonymised borrowers, covering a total of 16m transactions.
On top of this, we fed in loan information from a credit reference agency for another 4.6m anonymised individuals, covering 50m products purchased.
What’s been most interesting here though, from my perspective, is the potential that processing power now gives our economists to undertake the most sophisticated customer lifetime profitability analysis.
In other words, we can if we want to, mimic the modelling that firms themselves use to analyse their customers and, in turn, develop a sophisticated understanding of how individual businesses and markets are making their decisions. And, as it turns out, for many firms there’s been a willingness to make a loss on the first loan – on the basis that the hook has been dangled and, in all probability, that the customer will return to them for a second, third, fourth (or more) loans where the margins are greater (because the risk of customers defaulting on the loan are much smaller).
So we have been to build up a detailed picture here of borrowing patterns, costs and financial circumstances in this market. Some interesting results here. So, the analysis shows that, on average, payday customers are taking out some six loans a year. Those users also tend to be younger than the UK average. When you then apply this work in a regression discontinuity design model, you also see the differences in outcomes for similar consumers who were narrowly successful, or unsuccessful, in their pay-day loan application.
This creates a natural experiment with historical data, roughly equivalent to a randomised controlled trial, from which we can infer the causal effects of pay-day loan use.
For example, we were able to see that getting a pay-day loan made consumers just over 2% less likely to exceed their overdraft limit in the month of application (clearly because they had the money they borrowed) but this quickly reversed as they needed to repay the loan.
In fact, a few months in, they were 4% more likely to exceed their overdraft limit every month. a persistent long-run cost for a short-term loan. So, some highly important statistics here for policy makers. Yet arguably the most important step forward for regulators, has been what this data and processing power allows us to do next - in terms of testing potential interventions. In effect, offering us the possibility of running sophisticated simulations of the impact of alternative price caps on the market.
In other words, we can model what happens to borrowers as you raise or lower the price cap level, as well as monitor issues like firm exits; the viability of the market under different scenarios; and the potential risk of vulnerable people turning to unlicensed lenders. This, in turn of course, allows you to narrow down policy options more effectively and set a more authoritative cap level. In this case, the proposal is for a 0.8% initial cost cap per day, £15 default fee cap and total cost cap of 100%. Saving pay day consumers, on average, an estimated £180 a year or £157m in aggregate, while firms’ revenue is modelled to fall by some £220m a year.
Now, time will tell how accurate this kind of prediction work becomes. History hasn’t always flattered economists or their attempts to use technology to forecast the future. No-one wants to reproduce the 21st century equivalent of Irving Fisher’s economic predictor here, with its hydraulic bellows, levers, cisterns, floats and rods. But whether you agree with the fundamental principle of price caps or not, it’s frankly impossible to argue we’re not better placed to set them today than we were five years ago.
So, although I’m sure we’ll still see multiple debates over policy direction in future – and we’re still refining methods and working towards publishing more occasional papers in the new year – for me, there’s no doubt that today’s improvements in technology offer us some important possibilities. Not least in reducing the impact of unintended consequence.
In fact, I’d argue that, almost overnight, economic analysis, technology and data has become an imperative in terms of addressing that critical question: how do you make sure regulatory intervention becomes socially useful intervention? Not just mere ‘activity’.
And this is a priority that’s now dominating FCA policy formation across multiple areas, including our competition work in markets like general insurance add-ons; cash savings; and more recently on overdrafts.
GI add-ons
A few words on each, starting with GI-add ons, which we published a competition market study on in March this year - in response to wider concerns over how well the market was functioning. Key issues here: the fact that consumers were paying far more than the costs of providing many add-on products. Anecdotal evidence from consumer organisations that some of these products were being sold, rather than bought. A subtle but important difference.
Finally, and perhaps the most important potential issue with GI add-ons, the fact that our previous work in areas like mobile phone insurance had shown that these markets didn’t seem to be driving efficient consumer outcomes.
The study itself focused on whether there are significant challenges around how competition is working for consumers across the different markets for add-ons. Travel insurance cover offered alongside a holiday, for example, or GAP insurance with a new car and so on and so forth.
At the centre of this debate: are there any issues for firms over the actual mechanics of the add-on sale model? And, alongside this, some important questions that we wanted answered, including: how do consumers behave in these markets? And how does the add-on sales format influence their buying decisions? From the analytical perspective, what was interesting here was the way we combined new and traditional evidence to get to the bottom of how the market was really working.
So, yes, there was the normal financial analysis of firms and consumer research. But there was also the more novel use of experiments to generate additional insights. Some of our experimental findings were striking: when the price of add-on insurance was revealed at the point of buying the primary product, 65% of buyers purchased the first insurance they saw, compared with just 16% when sold separately, and 17% if the insurance was revealed and priced up-front alongside the primary product.
In other words, the later the price is shown, the less likely the customer is to shop around for alternatives. There seems to be a ‘point of no return’ if you will. Perhaps because people go so far they don’t want to go back and do it all over again. Perhaps because people go into mental ‘shut down’. They’ve already made the choice and don’t want to revisit the information.
The problem here is that this, in turn, led consumers to pay some 15% more when the price of the insurance was revealed later than if it was labelled up front. They were also more likely to make mistakes: 24% did not choose the cheapest overall bundle, compared to four per cent when consumers bought the insurance separately.
In fact the controlled experimental setting allowed us to dig deeper: it was not just the lack of transparency; revealing the price later that was driving these mistakes. We found that one in five consumers did not choose the cheapest bundle on the main and add-on product, even if both prices were presented up front. Even this relatively small increase in price complexity, having to add two prices together – proved a significant barrier to shopping around.
Now, for those of us who’ve been around financial services for any length of time, the trends here will not come as any great surprise – even if the numbers do. Most of us know and have seen the impact of complexity on decision making multiple times.
What has changed today however, as I mentioned earlier, is our approach to understanding of how it affects consumers in different markets, enabled by combining testing, behavioural economics and traditional market analysis techniques – and how we can regulate more effectively as a result.
Take mandated disclosure, for example. In the UK, when faced with a breakdown of price efficiency or rationality, the standard response pre-crisis was to provide more information and provide it more quickly.
If someone did not appreciate the risk of a product, we extended the description; if it looked too risky, we pushed people to the risk profile that allowed a sale. People were required to tick the boxes – that they had high-risk appetites; that they had read and understood the terms and conditions; and that the decision was their own, that it was a non-advised sale.
The obvious problem here of course, is that no-one reads those T&Cs. We simply trust in the good will of the firm delivering them. So, as Omri Ben-Shahar and Carl Schneider argue in their excellent book ‘More than you Wanted to Know’, as a means of reducing information asymmetries and reducing complexity, mandated disclosure often doesn’t work.
We saw this clearly in our add-on GI research a vast majority of consumers told us they were satisfied with the clarity and amount of information they got about their policy. Even though later they did no better than guessing randomly when quizzed on the basic facts about the policy they bought.
In fact, most people make choices by stripping information away; not adding ever more detail. And of course, exactly the same holds true in bank, insurance contracts and the like – many of which are now longer than Hamlet.
So, in the case of GI-add-ons, the means by which we handle challenges like inertia and complexity now look very different to those that came before. There’s no longer that reliance on the invisible hand to do all the leg work. Instead, interventions are guided by serious analytical research and data into what works in that particular market. And indeed, what doesn’t work.
So, among other areas we’re looking at: a ban on pre-ticked boxes to challenge consumer inertia; publication of claims ratios as a sunlight remedy for press, consumer organizations and regulators to spot poor value products; consumers confirming purchase of GAP insurance again after the sale; and improvements to the way add-ons are offered through price-comparison sites.
We are currently in the process of analysing literally millions of data points pulled in from the market, including detailed account and balance information; interest rates; distribution channels; switching patterns; disclosure documents and so on and so forth.
Cash savings
On the UK’s cash savings market, the second competition area I wanted to mention, a similarly sophisticated level of work is now underway following the launch of a market study in October last year. Now, we know some 82% of adults have a savings account. We also know a large number of firms compete to supply cash savings products.
But as we saw in July’s interim report, the data we hold shows there are clear issues here. The most important being the fact that the large majority of us pay little or no attention to alternative accounts on offer. We cling to what we have. We are currently in the process of analysing literally millions of data points pulled in from the market, including detailed account and balance information; interest rates; distribution channels; switching patterns; disclosure documents and so on and so forth.
Our behavioural economists have also been running a field trial with a firm to find out whether a well-timed reminder letter or other kind of communication changes consumer behaviour in relation to a decrease in their interest rate. That process has involved splitting thousands of consumers into several test groups, to analyse whether changes to information help real consumers in a real market environment achieve better outcomes.
If there are any behavioural economists here this evening, you probably won’t be surprised that the emerging results show the importance of behavioural insights in conducting market analysis. As our study on GI add-ons showed: understanding how consumers behave and how firms respond to this – how competition works, whether consumers are disciplining firms effectively – is the key to understanding markets. And understanding markets is the vital first step in regulating effectively.
Looking forward, we’ll be publishing the final cash savings report soon. But again, a significant opportunity here I think for regulators to use econometric techniques, technology and big data alongside behavioural economics to better understand how markets work.
Overdrafts
Finally, a few reflections on what’s rapidly become one of our most significant areas of policy formation work: the UK’s complex current account and overdraft market. Now it is quite inconceivable, frankly, that regulators could have developed a sophisticated-enough picture of the market ten years ago, to drive the quality of work we are currently able to undertake. To work out how new technology like mobile banking affects how consumers behave, what information they respond to, and what might efficiently reduce how often they hit unexpected charges.
So, in terms of raw data: we’ve been able to analyse the anonymised personal current account activity of some 500,000 customers, with more than 621,000 accounts between them. Collecting monthly information on transactions dating back to 2011.
What then does this data potentially allow us to do? Well, for starters, it gives us unprecedented richness of technical detail on the market. And that, in turn, opens up the prospect of deeper regulatory understanding of what’s driving consumer financial decisions and how receptive different types of consumers are to market events – including regulatory interventions.
As in the case of our pay cap work, this is the critical phase. Indeed, we have found considerable differences in the effectiveness of market initiatives to help consumers avoid unexpected charges, the annual statements regulatory initiative having zero impact while the mobile banking app effectively reduces overdraft charges. Now this understanding is important because it removes a lot of policy guesswork. You can refine and target regulation more effectively.
So, for example, we can begin to assess both the customers who are most susceptible to overdraft charges as well as those who forego the most potential interest from not switching.
On top of this, we’ve been able to disaggregate information to a customer (and a monthly basis) on everything from turnover and expenditure; unarranged and arranged overdraft charges (including the number of days spent in overdraft) to arranged overdraft limits; when customers started their account, cross product holdings including information on loans, credit cards and savings, demographic information and so on.
Now this may not sound too exciting to some, it certainly lacks the pizzazz of a big enforcement case, but for policy makers it’s actually enormously important. The regulatory equivalent of the leap from black and white to glorious technicolour.
Again, full details of our work here are not being published until early next year, to give us time to run regressions on some one billion data points. But in the meantime, it’s worth noting, I think, that this is now all part of a much broader pattern of FCA work. A useful example: the randomised controlled trials we are running to research how smarter disclosures in car and home insurance renewal letters impacts decision-making. In effect, we want to understand how consumers act in response to different types of information. To understand what makes disclosure effective or not.
For instance, might including information on last year’s insurance premium prompt people to consider moving to another provider? And how can we encourage consumers to use information to make better choices? Clearly, it’s important for disclosure work to evolve as new technology and research comes online.
We are, of course, keen to make this happen and we’re working with firms to help them communicate with customers more effectively – in fact we’re inviting firms to talk to us about testing new ideas or techniques that could improve customer engagement. As part of this, we are now able to waive rules more easily, such as the specifics of disclosure requirements, to allow testing that could produce more effective regulatory solutions. This work is all wrapped up under the FCA’s Project Innovate, which is encouraging firms and their advisers to work with us on trials that may, potentially, benefit both themselves and their customers.
It is, frankly, impossible to guarantee success at all times. But behavioural economics, data and technology do offer policy makers the possibility that, in future, far more regulatory action can have a meaningful and positive societal contribution. In other word, economics has never been more important to the future of our financial services or its customers.
Conclusion
So, as I say, some significant insights now being generated by the huge strides forward we have seen in technology and data – coupled with that shift in regulatory philosophy towards understanding and regulating markets of real consumers. Already, we are seeing some encouraging winds of change in the system as a result. Insurance companies reducing disclosure. Change programmes across industry. Banks simplifying terms & conditions and using teaser rates more judiciously. More work remains to be done. But for me, the key issue moving forward is for global regulators to embrace modern, 21st century economics to try and remove the guesswork from interventions.
It is, frankly, impossible to guarantee success at all times. But behavioural economics, data and technology do offer policy makers the possibility that, in future, far more regulatory action can have a meaningful and positive societal contribution. In other word, economics has never been more important to the future of our financial services or its customers.